What is machine learning and how does it work? In-depth guide
Machine learning (ML) is a type of artificial intelligence (AI) focused on building computer systems that learn from data. The broad range of techniques ML encompasses enables software applications to improve their performance over time.
Machine learning algorithms are trained to find relationships and patterns in data. They use historical data as input to make predictions, classify information, cluster data points, reduce dimensionality and even help generate new content, as demonstrated by new ML-fueled applications such as ChatGPT, Dall-E 2 and GitHub Copilot.
Machine learning is widely applicable across many industries. Recommendation engines, for example, are used by e-commerce, social media and news organizations to suggest content based on a customer's past behavior. Machine learning algorithms and machine vision are a critical component of self-driving cars, helping them navigate the roads safely. In healthcare, machine learning is used to diagnose and suggest treatment plans. Other common ML use cases include fraud detection, spam filtering, malware threat detection, predictive maintenance and business process automation.
While machine learning is a powerful tool for solving problems, improving business operations and automating tasks, it's also a complex and challenging technology, requiring deep expertise and significant resources. Choosing the right algorithm for a task calls for a strong grasp of mathematics and statistics. Training machine learning algorithms often involves large amounts of good quality data to produce accurate results. The results themselves can be difficult to understand -- particularly the outcomes produced by complex algorithms, such as the deep learning neural networks patterned after the human brain. And ML models can be costly to run and tune.
Still, most organizations either directly or indirectly through ML-infused products are embracing machine learning. According to the "2023 AI and Machine Learning Research Report" from Rackspace Technology, 72% of companies surveyed said that AI and machine learning are part of their IT and business strategies, and 69% described AI/ML as the most important technology. Companies that have adopted it reported using it to improve existing processes (67%), predict business performance and industry trends (60%) and reduce risk (53%).
TechTarget's guide to machine learning is a primer on this important field of computer science, further explaining what machine learning is, how to do it and how it is applied in business. You'll find information on the various types of machine learning algorithms, the challenges and best practices associated with developing and deploying ML models, and what the future holds for machine learning. Throughout the guide, there are hyperlinks to related articles that cover the topics in greater depth.
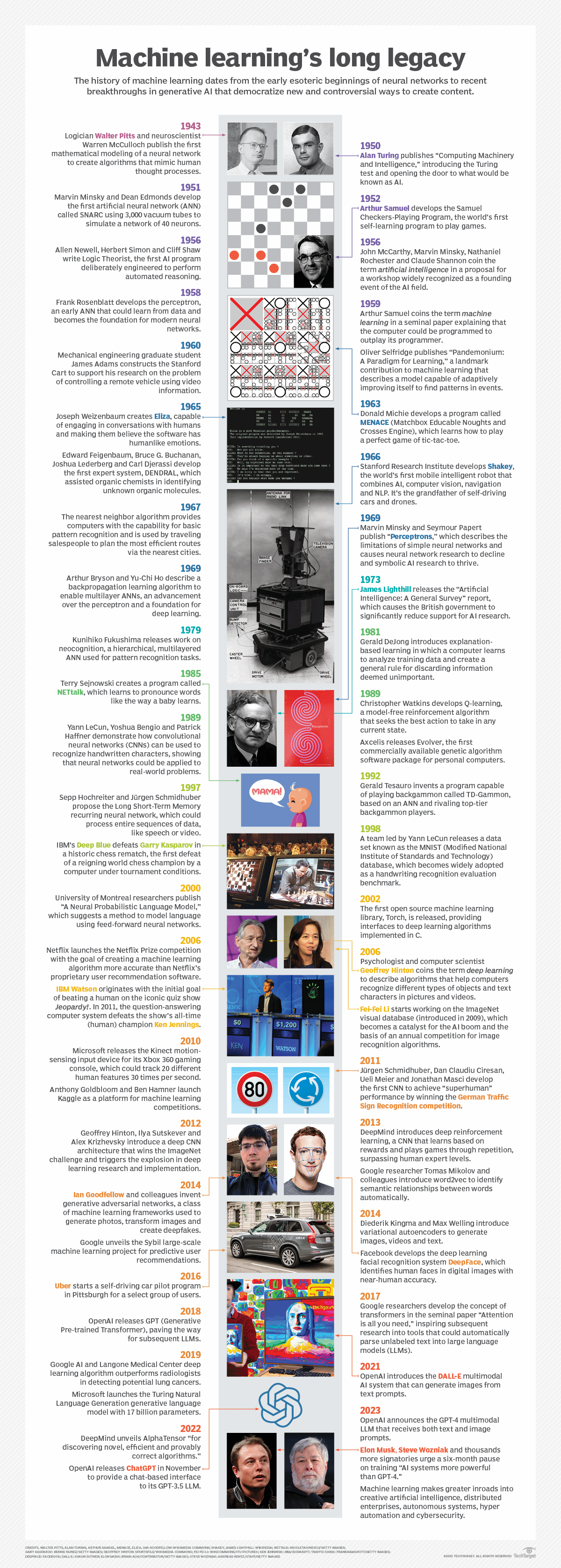
Why is machine learning important?
Machine learning has played a progressively central role in human society since its beginnings in the mid-20th century, when AI pioneers like Walter Pitts, Warren McCulloch, Alan Turing and John von Neumann laid the groundwork for computation. The training of machines to learn from data and improve over time has enabled organizations to automate routine tasks that were previously done by humans -- in principle, freeing us up for more creative and strategic work.
Machine learning also performs manual tasks that are beyond our ability to execute at scale -- for example, processing the huge quantities of data generated today by digital devices. Machine learning's ability to extract patterns and insights from vast data sets has become a competitive differentiator in fields ranging from finance and retail to healthcare and scientific discovery. Many of today's leading companies, including Facebook, Google and Uber, make machine learning a central part of their operations.
As the volume of data generated by modern societies continues to proliferate, machine learning will likely become even more vital to humans and essential to machine intelligence itself. The technology not only helps us make sense of the data we create, but synergistically the abundance of data we create further strengthens ML's data-driven learning capabilities.
What will come of this continuous learning loop? Machine learning is a pathway to artificial intelligence, which in turn fuels advancements in ML that likewise improve AI and progressively blur the boundaries between machine intelligence and human intellect.
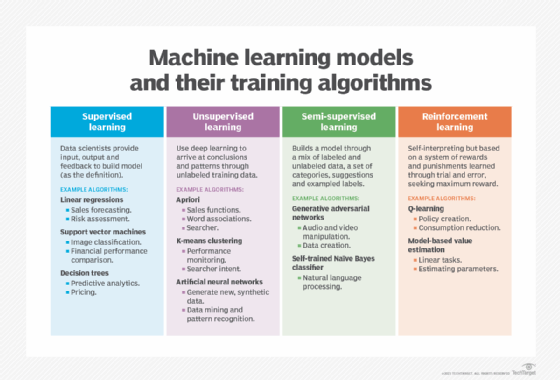
What are the different types of machine learning?
Classical machine learning is often categorized by how an algorithm learns to become more accurate in its predictions. There are four basic types of machine learning: supervised learning, unsupervised learning, semisupervised learning and reinforcement learning.
The type of algorithm data scientists choose depends on the nature of the data. Many of the algorithms and techniques aren't limited to just one of the primary ML types listed here. They're often adapted to multiple types, depending on the problem to be solved and the data set. For instance, deep learning algorithms such as convolutional neural networks and recurrent neural networks are used in supervised, unsupervised and reinforcement learning tasks, based on the specific problem and availability of data.
Machine learning vs. deep learning neural networks
Deep learning is a subfield of ML that deals specifically with neural networks containing multiple levels -- i.e., deep neural networks. Deep learning models can automatically learn and extract hierarchical features from data, making them effective in tasks like image and speech recognition.
How does supervised machine learning work?
In supervised learning, data scientists supply algorithms with labeled training data and define the variables they want the algorithm to assess for correlations. Both the input and output of the algorithm are specified in supervised learning. Initially, most machine learning algorithms worked with supervised learning, but unsupervised approaches are becoming popular.
Supervised learning algorithms are used for several tasks, including the following:
- Binary classification. Divides data into two categories.
- Multiclass classification. Chooses between more than two types of answers.
- Ensembling. Combines the predictions of multiple ML models to produce a more accurate prediction.
- Regression modeling. Predicts continuous values based on relationships within data.
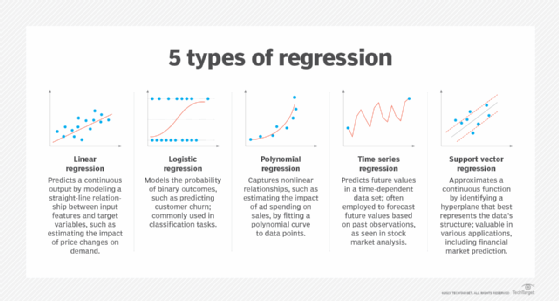
How does unsupervised machine learning work?
Unsupervised machine learning algorithms don't require data to be labeled. They sift through unlabeled data to look for patterns that can be used to group data points into subsets. Most types of deep learning, including neural networks, are unsupervised algorithms.
Unsupervised learning algorithms are good for the following tasks:
- Clustering. Splitting the data set into groups based on similarity using clustering algorithms.
- Anomaly detection. Identifying unusual data points in a data set using anomaly detection algorithms.
- Association rule. Discovering sets of items in a data set that frequently occur together using association rule mining.
- Dimensionality reduction. Decreasing the number of variables in a data set using dimensionality reduction techniques.
How does semisupervised learning work?
Semisupervised learning works by feeding a small amount of labeled training data to an algorithm. From this data, the algorithm learns the dimensions of the data set, which it can then apply to new unlabeled data. The performance of algorithms typically improves when they train on labeled data sets. But labeling data can be time-consuming and expensive. This type of machine learning strikes a balance between the superior performance of supervised learning and the efficiency of unsupervised learning.
Semisupervised learning can be used in the following areas, among others:
- Machine translation. Teaches algorithms to translate language based on less than a full dictionary of words.
- Fraud detection. Identifies cases of fraud when there are only a few positive examples.
- Labeling data. Algorithms trained on small data sets learn to apply data labels to larger sets automatically.
How does reinforcement learning work?
Reinforcement learning works by programming an algorithm with a distinct goal and a prescribed set of rules for accomplishing that goal. A data scientist will also program the algorithm to seek positive rewards for performing an action that's beneficial to achieving its ultimate goal and to avoid punishments for performing an action that moves it farther away from its goal.
Reinforcement learning is often used in the following areas:
- Robotics. Robots learn to perform tasks in the physical world.
- Video gameplay. Teaches bots to play video games.
- Resource management. Helps enterprises plan allocation of resources.
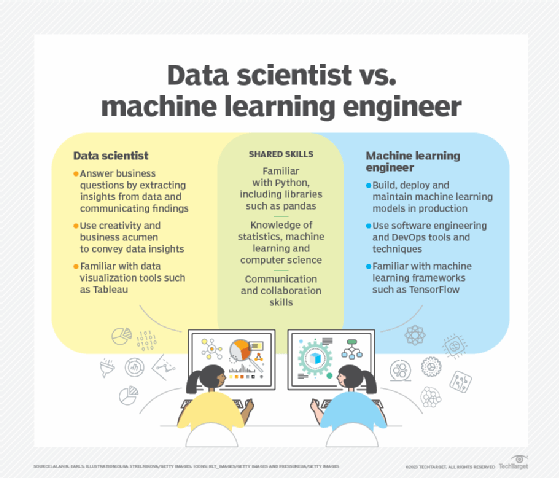
How to choose and build the right machine learning model
Developing the right machine learning model to solve a problem can be complex. It requires diligence, experimentation and creativity, as detailed in a seven-step plan on how to build an ML model, a summary of which follows.
1. Understand the business problem and define success criteria. The goal is to convert the group's knowledge of the business problem and project objectives into a suitable problem definition for machine learning. Questions should include why the project requires machine learning, what type of algorithm is the best fit for the problem, whether there are requirements for transparency and bias reduction, and what the expected inputs and outputs are.
2. Understand and identify data needs. Determine what data is necessary to build the model and whether it's in shape for model ingestion. Questions should include how much data is needed, how the collected data will be split into test and training sets, and if a pre-trained ML model can be used.
3. Collect and prepare the data for model training. Actions include cleaning and labeling the data; replacing incorrect or missing data; enhancing and augmenting data; reducing noise and removing ambiguity; anonymizing personal data; and splitting the data into training, test and validation sets.
4. Determine the model's features and train it. Select the right algorithms and techniques. Set and adjust hyperparameters, train and validate the model, and then optimize it. Depending on the nature of the business problem, machine learning algorithms can incorporate natural language understanding capabilities, such as recurrent neural networks or transformers that are designed for NLP tasks. Additionally, boosting algorithms can be used to optimize decision tree models.
Training and optimizing ML models
Learn how the following algorithms and techniques are used in training and optimizing machine learning models:
5. Evaluate the model's performance and establish benchmarks. The work here encompasses confusion matrix calculations, business key performance indicators, machine learning metrics, model quality measurements and determining whether the model can meet business goals.
6. Deploy the model and monitor its performance in production. This part of the process is known as operationalizing the model and is typically handled collaboratively by data science and machine learning engineers. Continually measure the model for performance, develop a benchmark against which to measure future iterations of the model and iterate to improve overall performance. Deployment environments can be in the cloud, at the edge or on the premises.
7. Continuously refine and adjust the model in production. Even after the ML model is in production and continuously monitored, the job continues. Business requirements, technology capabilities and real-world data change in unexpected ways, potentially giving rise to new demands and requirements.
Machine learning applications for enterprises
Machine learning has become integral to the business software that runs organizations. The following are some examples of how various disciplines use ML:
- Business intelligence. BI and predictive analytics software use machine learning algorithms, including linear regression and logistic regression, to identify significant data points, patterns and anomalies in large data sets.
- Customer relationship management. Key applications of machine learning in CRM include analyzing customer data to segment customers, predicting behaviors such as churn, making recommendations, adjusting pricing, optimizing email campaigns, providing chatbot support and detecting fraud.
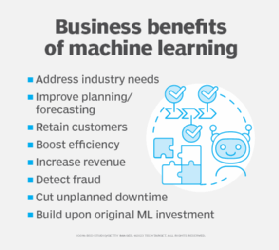
- Security and compliance. Advanced algorithms -- such as anomaly detection and support vector machine (SVM) techniques -- identify normal behavior and deviations, which is crucial in identifying potential cyberthreats. SVMs find the best line or boundary that divides data into different groups separated by as much space as possible.
- Human resource information systems. ML models streamline the hiring process by filtering through applications and identifying the best candidates for an open position.
- Supply chain management. Machine learning techniques optimize inventory levels, streamline logistics, improve supplier selection and proactively address supply chain disruptions.
- Natural language processing. ML models enable virtual assistants like Alexa, Google Assistant and Siri to interpret and respond to human language.
What are the advantages and disadvantages of machine learning?
Machine learning's ability to identify trends and predict outcomes with higher accuracy than methods that rely strictly on conventional statistics -- or human intelligence -- provides a competitive advantage to businesses that deploy ML effectively. Machine learning can benefit businesses in several ways:
- Analyzing historical data to retain customers.
- Launching recommender systems to grow revenue.
- Improving planning and forecasting.
- Assessing patterns to detect fraud.
- Boosting efficiency and cutting costs.
But machine learning also comes with disadvantages. First and foremost, it can be expensive. Machine learning projects are typically driven by data scientists, who command high salaries. These projects also require software infrastructure that can be expensive. And businesses can encounter many more challenges.
There's the problem of machine learning bias. Algorithms trained on data sets that exclude certain populations or contain errors can lead to inaccurate models of the world that, at best, fail and, at worst, are discriminatory. When an enterprise bases core business processes on biased models, it can suffer regulatory and reputational harm.
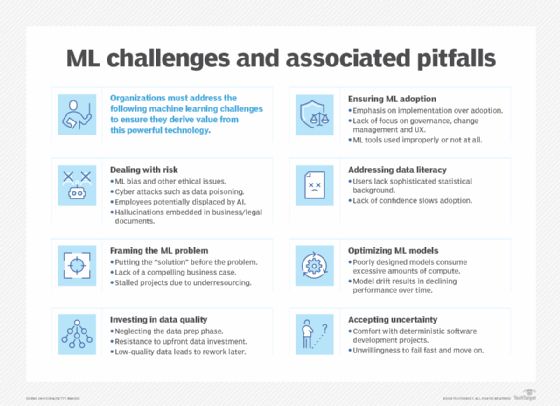
Importance of human-interpretable machine learning
Explaining how a specific ML model works can be challenging when the model is complex. In some vertical industries, data scientists must use simple machine learning models because it's important for the business to explain how every decision was made. That's especially true in industries that have heavy compliance burdens, such as banking and insurance. Data scientists often find themselves having to strike a balance between transparency and the accuracy and effectiveness of a model. Complex models can produce accurate predictions, but explaining to a layperson -- or even an expert -- how an output was determined can be difficult.
Careers in machine learning and AI
The global AI market's value is expected to reach nearly $2 trillion by 2030, and the need for skilled AI professionals is growing in kind. Check out the following articles related to ML and AI professional development.
How to build and organize a machine learning team
Prep with 19 machine learning interview questions and answers
Machine learning examples in industry
Machine learning has been widely adopted across industries. Here are some of the sectors using machine learning to meet their market requirements:
- Financial services. Risk assessment, algorithmic trading, customer service and personalized banking are areas where financial services companies apply machine learning. Capital One, for example, deployed ML for credit card defense, which the company places in the broader category of anomaly detection.
- Pharmaceuticals. Drug makers use ML for drug discovery, in clinical trials and in drug manufacturing. Eli Lilly has built AI and ML models, for example, to find the best sites for clinical trials and boost the diversity of participants. The models have sharply reduced clinical trial timelines, according to the company.
- Manufacturing. Predictive maintenance use cases are prevalent in the manufacturing industry, where an equipment breakdown can lead to expensive production delays. In addition, the computer vision aspect of machine learning can inspect items coming off a production line to ensure quality control.
- Insurance. Recommendation engines can suggest options for clients based on their needs and how other customers have benefited from specific insurance products. Machine learning is also useful in underwriting and claims processing.
- Retail. In addition to recommendation systems, retailers use computer vision for personalization, inventory management and planning the styles and colors of a given fashion line. Demand forecasting is another key use case.
What is the future of machine learning?
Fueled by the massive amount of research by companies, universities and governments around the globe, machine learning is a rapidly moving target. Breakthroughs in AI and ML seem to happen daily, rendering accepted practices obsolete almost as soon as they're accepted. One thing that can be said with certainty about the future of machine learning is that it will continue to play a central role in the 21st century, transforming how work gets done and the way we live.
In the field of NLP, improved algorithms and infrastructure will give rise to more fluent conversational AI, more versatile ML models capable of adapting to new tasks and customized language models fine-tuned to business needs.
The fast-evolving field of computer vision is expected to have a profound effect on many domains, from healthcare where it will play an increasingly important role in diagnosis and monitoring as the technology improves, to environmental science where it could be used to analyze and monitor habitats, to software engineering where it's a core component of augmented and virtual reality technologies.
In the near term, machine learning platforms are among enterprise technology's most competitive realms. Major vendors like Amazon, Google, Microsoft, IBM and OpenAI are racing to sign customers up for automated machine learning platform services that cover the spectrum of ML activities, including data collection, data preparation, data classification, model building, training and application deployment.
Amid the enthusiasm, companies will face many of the same challenges presented by previous cutting-edge, fast-evolving technologies. New challenges include adapting legacy infrastructure to machine learning systems, mitigating ML bias and figuring out how to best use these awesome new powers of AI to generate profits for enterprises, in spite of the costs.