recurrent neural networks
What are recurrent neural networks?
A recurrent neural network is a type of artificial neural network commonly used in speech recognition and natural language processing. Recurrent neural networks recognize data's sequential characteristics and use patterns to predict the next likely scenario.
RNNs are used in deep learning and in the development of models that simulate neuron activity in the human brain. They are especially powerful in use cases where context is critical to predicting an outcome, and are also distinct from other types of artificial neural networks because they use feedback loops to process a sequence of data that informs the final output. These feedback loops allow information to persist. This effect often is described as memory.
RNN use cases tend to be connected to language models in which knowing the next letter in a word or the next word in a sentence is predicated on the data that comes before it. A compelling experiment involves an RNN trained with the works of Shakespeare to produce Shakespeare-like prose successfully. Writing by RNNs is a form of computational creativity. This simulation of human creativity is made possible by the AI's understanding of grammar and semantics learned from its training set.
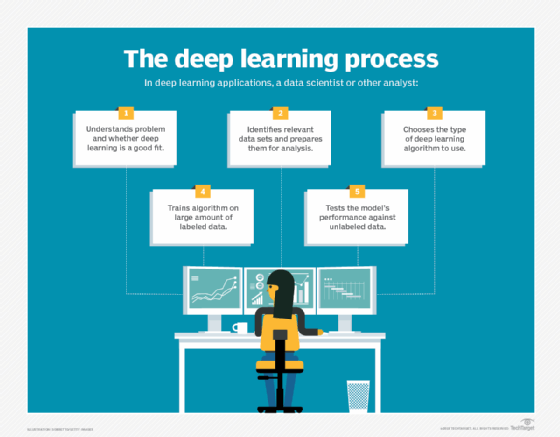
How recurrent neural networks learn
Artificial neural networks are created with interconnected data processing components that are loosely designed to function like the human brain. They are composed of layers of artificial neurons -- network nodes -- that have the ability to process input and forward output to other nodes in the network. The nodes are connected by edges or weights that influence a signal's strength and the network's ultimate output.
In some cases, artificial neural networks process information in a single direction from input to output. These "feed-forward" neural networks include convolutional neural networks that underpin image recognition systems. RNNs, on the other hand, can be layered to process information in two directions.
Like feed-forward neural networks, RNNs can process data from initial input to final output. Unlike feed-forward neural networks, RNNs use feedback loops, such as backpropagation through time, throughout the computational process to loop information back into the network. This connects inputs and is what enables RNNs to process sequential and temporal data.
A truncated backpropagation through time neural network is an RNN in which the number of time steps in the input sequence is limited by a truncation of the input sequence. This is useful for recurrent neural networks that are used as sequence-to-sequence models, where the number of steps in the input sequence (or the number of time steps in the input sequence) is greater than the number of steps in the output sequence.
Bidirectional recurrent neural networks
Bidirectional recurrent neural networks (BRNNs) are another type of RNN that simultaneously learn the forward and backward directions of information flow. This is different from standard RNNs, which only learn information in one direction. The process of both directions being learned simultaneously is known as bidirectional information flow.
In a typical artificial neural network, the forward projections are used to predict the future, and the backward projections are used to evaluate the past. They are not used together, however, as in a BRNN.
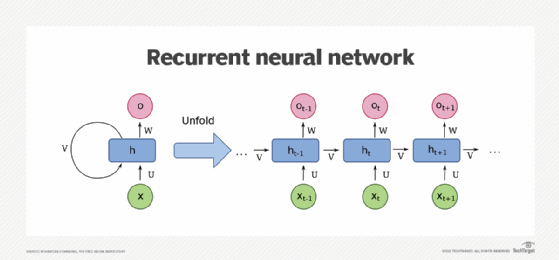
RNN challenges and how to solve them
The most common issues with RNNS are gradient vanishing and exploding problems. The gradients refer to the errors made as the neural network trains. If the gradients start to explode, the neural network will become unstable and unable to learn from training data.
Long short-term memory units
One drawback to standard RNNs is the vanishing gradient problem, in which the performance of the neural network suffers because it can't be trained properly. This happens with deeply layered neural networks, which are used to process complex data.
Standard RNNs that use a gradient-based learning method degrade as they grow bigger and more complex. Tuning the parameters effectively at the earliest layers becomes too time-consuming and computationally expensive.
One solution to the problem is called long short-term memory (LSTM) networks, which computer scientists Sepp Hochreiter and Jurgen Schmidhuber invented in 1997. RNNs built with LSTM units categorize data into short-term and long-term memory cells. Doing so enables RNNs to figure out which data is important and should be remembered and looped back into the network. It also enables RNNs to figure out what data can be forgotten.
Gated recurrent units
Gated recurrent units (GRUs) are a form of recurrent neural network unit that can be used to model sequential data. While LSTM networks can also be used to model sequential data, they are weaker than standard feed-forward networks. By using an LSTM and a GRU together, networks can take advantage of the strengths of both units -- the ability to learn long-term associations for the LSTM and the ability to learn from short-term patterns for the GRU.
Multilayer perceptrons and convolutional neural networks
The other two types of classes of artificial neural networks include multilayer perceptrons (MLPs) and convolutional neural networks.
MLPs consist of several neurons arranged in layers and are often used for classification and regression. A perceptron is an algorithm that can learn to perform a binary classification task. A single perceptron cannot modify its own structure, so they are often stacked together in layers, where one layer learns to recognize smaller and more specific features of the data set.
The neurons in different layers are connected to each other. For example, the output of the first neuron is connected to the input of the second neuron, which acts as a filter. MLPs are used to supervise learning and for applications such as optical character recognition, speech recognition and machine translation.
Convolutional neural networks, also known as CNNs, are a family of neural networks used in computer vision. The term "convolutional" refers to the convolution -- the process of combining the result of a function with the process of computing/calculating it -- of the input image with the filters in the network. The idea is to extract properties or features from the image. These properties can then be used for applications such as object recognition or detection.
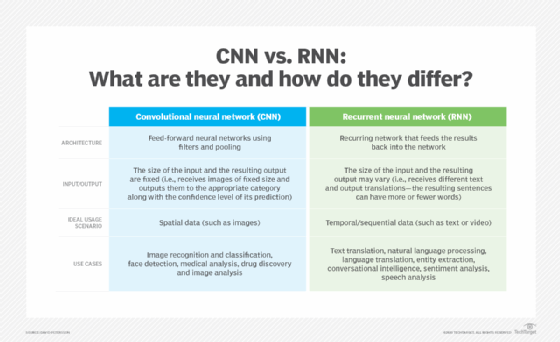
CNNs are created through a process of training, which is the key difference between CNNs and other neural network types. A CNN is made up of multiple layers of neurons, and each layer of neurons is responsible for one specific task. The first layer of neurons might be responsible for identifying general features of an image, such as its contents (e.g., a dog). The next layer of neurons might identify more specific features (e.g., the dog's breed).