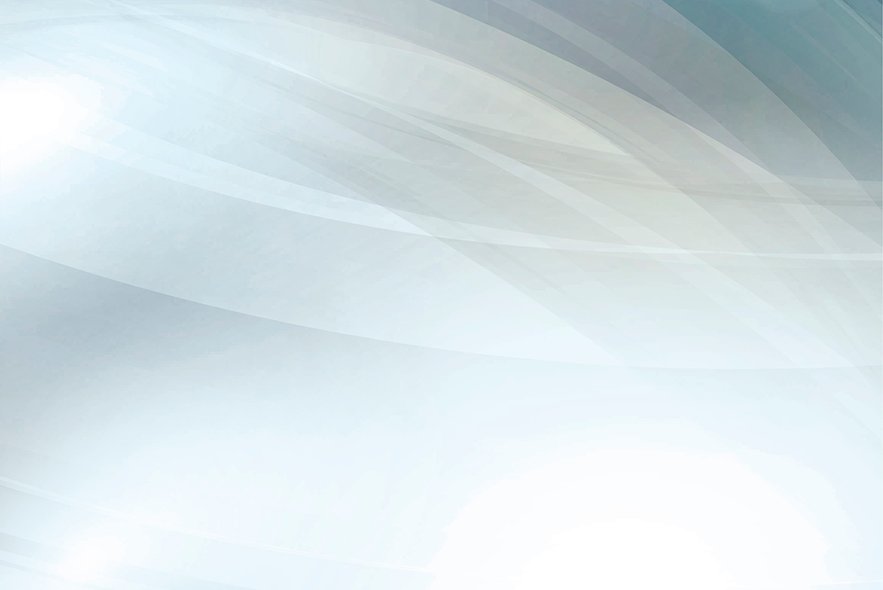
machine learning bias (AI bias)
What is machine learning bias (AI bias)?
Machine learning bias, also known as algorithm bias or AI bias, is a phenomenon that occurs when an algorithm produces results that are systemically prejudiced due to erroneous assumptions in the machine learning (ML) process.
Machine learning, a subset of artificial intelligence (AI), depends on the quality, objectivity and size of training data used to teach it. Faulty, poor or incomplete data results in inaccurate predictions, reflecting the garbage in, garbage out admonishment used in computer science to convey the concept that the quality of the output is determined by the quality of the input.
Machine learning bias generally stems from problems introduced by the individuals who design and train the machine learning systems. These people could either create algorithms that reflect unintended cognitive biases or real-life prejudices. Or they could introduce biases because they use incomplete, faulty or prejudicial data sets to train and validate the machine learning systems.
Types of cognitive bias that can inadvertently affect algorithms include stereotyping, the bandwagon effect, priming, selective perception and confirmation bias.
Although these biases are often unintentional, the consequences of their presence in ML systems can be significant. Depending on how the machine learning systems are used, such biases could result in bad customer service experiences, reduced sales and revenue, unfair or possibly illegal actions, and potentially dangerous conditions.
To prevent such scenarios, organizations should check the data being used to train machine learning models for lack of comprehensiveness and cognitive bias. The data should be representative of different races, genders, backgrounds and cultures that could be adversely affected. Data scientists developing the algorithms should shape data samples in a way that minimizes algorithmic and other types of machine learning bias, and decision-makers should evaluate when it's appropriate, or inappropriate, to apply ML technology.
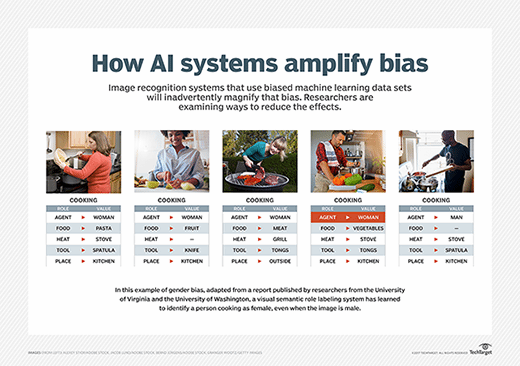
Types of machine learning bias
There are various ways that bias can be brought into a machine learning system. Common scenarios, or types of bias, include the following:
- Algorithm bias. This occurs when there's a problem within the algorithm that performs the calculations that power the machine learning computations.
- Sample bias. This happens when there's a problem with the data used to train the machine learning model. In this type of bias, the data used either isn't large enough or representative enough to teach the system. For example, using training data that features only female teachers trains the system to conclude that all teachers are female.
- Prejudice bias. In this case, the data used to train the system reflects existing prejudices, stereotypes and faulty societal assumptions, thereby introducing those same real-world biases into the machine learning itself. For example, using data about medical professionals that includes only female nurses and male doctors could perpetuate a real-world gender stereotype about healthcare workers in the computer system.
- Measurement bias. As the name suggests, this bias arises due to underlying problems with the accuracy of the data and how it was measured or assessed. Using pictures of happy workers to train a system meant to assess a workplace environment could be biased if the workers in the pictures knew they were being measured for happiness; a system being trained to precisely assess weight is biased if the weights contained in the training data were consistently rounded up.
- Exclusion bias. This happens when an important data point is left out of the data being used --something that can happen if the modelers don't recognize the data point as consequential.
- Selection bias. This occurs when the data used in training either isn't large enough or representative enough, thereby misrepresenting and lowering accuracy results and performance.
- Recall bias. This bias develops in the data labeling stage, where labels are inconsistently given through subjective observations. Recall is measured as how many points are labeled accurately over the total number of observations in a model.
Bias vs. variance
Data scientists and others involved in building, training and using ML models must consider not just bias, but also variance when seeking to create systems that can deliver consistently accurate results.
Like bias, variance is an error that results when machine learning produces the wrong assumptions based on the training data. Unlike bias, variance is a reaction to real and legitimate fluctuations in the data sets. These fluctuations, or noise, shouldn't affect the intended model, yet the system might still use that noise for modeling. In other words, variance is a problematic sensitivity to small fluctuations in the training set, which, like bias, can produce inaccurate results.
Although bias and variance are different, they're interrelated in that a level of variance can help reduce bias and a level of bias can reduce variance. If the data population has enough variety, biases should be drowned out by the variance.
As such, the objective in machine learning is to have a tradeoff, or balance, between the two in order to develop a system that produces a minimal amount of errors.
How to prevent bias
Awareness and governance can help prevent machine learning bias. An organization that recognizes the potential for bias can implement and institute best practices to combat it that include the following steps:
- Select training data that's appropriately representative and large enough to counteract common types of machine learning bias, such as sample and prejudice bias.
- Test and validate to ensure the results of machine learning systems don't reflect bias due to algorithms or data sets.
- Monitor ML systems as they perform their tasks to ensure biases don't creep in over time, as the systems continue to learn as they work.
- Use additional resources, such as Google's What-If Tool or IBM's AI Fairness 360 open source toolkit, to examine and inspect models.
- Create a data gathering method that accounts for different opinions. One data point could have multiple valid options for labels. When initially gathering data, taking those options into account increases the model's flexibility.
- Understand any training data used, as these training data sets could contain classes or labels that can introduce bias.
- Continually review the ML model, and plan to make improvements as more feedback is received.
History of machine learning bias
The term algorithmic bias was first defined by Trishan Panch and Heather Mattie in a program at the Harvard T.H. Chan School of Public Health. Machine learning bias has been a known risk for decades, yet it remains a complex problem that has been difficult to counteract.
In fact, machine learning bias has already been implicated in real-world cases, with some bias having significant and even life-altering consequences.
COMPAS is one such example. The COMPAS algorithm -- short for the Correctional Offender Management Profiling for Alternative Sanctions -- used machine learning to predict the potential for criminal defendants to reoffend. Multiple states had rolled out the software in the early part of the 21st century before its bias against people of color was exposed and subsequently publicized in news articles.
Amazon, a hiring powerhouse whose recruiting policies shape those at other companies, in 2018, scrapped its recruiting algorithm after it found that it was identifying word patterns. Rather than relevant skill sets, the algorithm inadvertently penalized resumes containing certain words, including women's -- a bias that favored male candidates over women candidates by discounting women's resumes.
Meanwhile, that same year, academic researchers announced findings that commercial facial recognition AI systems contained gender and skin-type biases.
Machine learning bias has also appeared in the medical field. For example, in 2019, a study unveiled that racial bias was found in an AI-based system that decided which patients need care in multiple hospitals. The AI algorithm showed racial bias, as black patients were labeled as being sicker than white patients recommended for the same care.
In a 2021 report from The Markup, AI bias was responsible for 80% of black mortgage applicants being denied. Likewise, lenders were 40% more likely to turn down Latino applicants, 50% more likely to turn down Asian/Pacific Islander applicants and 70% more likely to turn down Native American applicants, all when compared to similar white applicants.
Learn more about ways to reduce machine learning bias in its different forms.